 |
Simulating urban growth by two state modeling and connected network |
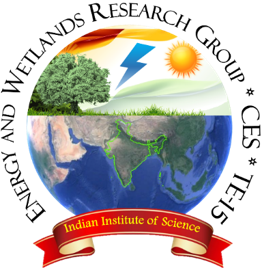 |
Methodology Adopted
Analysis can be delineated into five major steps (1) data acquisition from public repositories such as USGS, land use classification and accuracy assessment using open source software GRASS. (2) Land use analysis and baseline vali-dation. (3) Developing data for three scenarios based on urban growth. (4) Calibrating the model to find out the best weights based on the influence on the neighbourhood pix-els. (5) Development of model and validation of the model with predicting of future scenario based on validated data
and calculation of spatial metric for analysis of landscape
configuration in various scenarios.
1. Image pre-processing Remote sensing data were
acquired for specific years from USGS earth explorer
(http://www.usgs.gov/). Remotely sensing data obtained
were geo-referenced, rectified and cropped pertaining to
the study area.
2. Land use analysis Land use analysis was performed using
supervised pattern classifier—Gaussian maximum likeli-
hood classifier (GMLC). GMLC has been already proved
as one of the superior classification techniques due to use
of cost function and probability determination techniques
employed (Duda et al. 2000; Bharath et al. 2018). Land
use classification was performed and the classified data is
categorised into four major classes built-up area, vegeta-
tion, open area, and water body as described in Table 2.
Essentially land use analysis is performed in four
broad steps as follows.
a. Stacking as image composite-false colour composite
to identify various classes of patches (bands–near
infrared, green and red green).
b. Collection of training data as training polygons
using pre-calibrated GPS AND and using google
earth (validated and corrected with a shift in posi
tion). Training data was collected in order to clas-
sify and also to validate the results of the classifica
tion. 70% of the training data collected were used to
derive the user-classified map. 30% of exact ground
truth was used to validate it.
c. Land use classification using GMLC using GRASS
GIS (Geographical Analysis Support System) an
open source software has been used for the
analysis, which has the robust support for processing both
vector and raster files.
d. Validation of land use by performing accuracy
assessment and kappa statistics: accuracy assess-
ments helps the data generators to determine the
quality of the information. The test samples from
user classified map and validation ground truth maps
generated is use to generate well-known methods in
validation of land use using error matrix and calcu-
late kappa (κ) statistics.
3. Population growth rate has been a major influencing
factor of urban growth in Bangalore. Hence using this
as a factor three scenario was designed as per the suit-
ability map. The first scenario considered current growth
population rate of Bangalore (approach. 5% per annum)
as business as usual scenario, further with the national
growth rate at 2–3%, were considered as deviations for
designing the next two scenarios. The second scenario
was designed based on a decreased growth rate of 3%
matching the national average and the third scenario was
based on increased growth rate of 7%.
4. Modelling land use scenarios GeoMod was used for
modelling the land use pattern and to predict the future
scenarios. GeoMod is built on a grid-based model that
persists the maps as grids of data to simulate the urban
pattern of land use change and has a capability to predict
both as time forward or time backwards (Pontius and
Schneider 2001; Pontius et al. 2001; Dushku and Brown
2003). GeoMod works on a binary map and simulates
the land use change based on this binary map as two
categories (binary images are first created as urban and
non-urban). Map considered as a base to predict is supplied by the user and map to be used as validation is
also used (in case of validation) else only the grid cells
are mentioned, along with the land use change driver’s
associated with weights. This grid are assigned as one
of the two categories for the ending time based on the
various decision rules.
a. Map-persistence GeoMod can simulate two change
but in different transitions. In a single transition, it
simulates only a single way change.
b. Grid based differentiation or stratification It would
simulate land use change within a strata or specific
region.
c. Neighborhood constraint It is based on a nearest
neighbor principle and treats cells restrictions for
one time change with the edge between two portions
A&B in the land use.
d. Site suitability This is generated empirically using
several maps and the land-use transition map from
the beginning time.
Site suitability of each cell is calculated using the
equation below
where: R(i) = suitability value in cell (i), a = particular
driver map, A = the number of driver maps, Wa = the
weight of driver map a, and Pa (i) = percent-developed
in category ak of attribute map a, where cell (i) is a
member of category ak.
Figure 2 describes the identified drivers that have a
weighing influence on urbanization and process of mod-
elling and validation. Weightages were derived based on
the influence of each driver (Road, availability of public
transport etc.,) in urbanizing the neighbourhood pixels
through a multi criteria evaluation to derive a transition
suitability map.
5. Developed suitability map along in conjunction with
land use maps of the year 2008 and 2010 was considered
as base data to simulate urban growth in the year 2012.
The model performance was assessed by comparing the
simulated year 2012 urban map to the actual year 2012
classified map. Accuracy is calculated for each analysis.
This process is reiterated until the simulation reaches the
threshold accuracy by changing the driver behavior and
influence characteristics. Once the model is trained to
land use of 2010 and 2012 is used as base data to predict
urban pattern growth for the year 2014 to the year 2020
in a time step of 2 years and land use is quantified.
Spatial metrics using open source software fragstat
was then computed. This was performed for all scenarios
to observe the change in urban land use by 2020 depend-
ing land use configuration. As suggested by Ramachan-
dra and Bharath (2012) urban land use dynamics can
be characterized by few spatial metrics as tabulated in
Table 3 based on shape, edge, complexity criteria.
|
Citation :Bharath H. Aithal, S. Vinay, T. V. Ramachandra, 2018. Simulating urban growth by two state modelling and connected network. Modeling Earth Systems and Environment. © Springer Nature Switzerland AG 2018. Received: 30 April 2018 / Accepted: 11 August 2018. https://doi.org/10.1007/s40808-018-0506-1
|
Bharath.H Aithal RCG School of Infrastructure Design and Management, IIT Kharagpur, West Bengal, India
Energy and Wetland Research Group, Centre for Ecological Science, IISc, Bangalore, India
S.Vinay Energy and Wetland Research Group, Centre for Ecological Science, IISc, Bangalore, India
Dr. T.V. Ramachandra
Centre for Sustainable Technologies, Centre for infrastructure, Sustainable Transportation and Urban Planning (C iSTUP),
Energy & Wetlands Research Group, Centre for Ecological Sciences, Indian Institute of Science, Bangalore – 560 012, INDIA.
E-mail : cestvr@ces.iisc.ernet.in
Tel: 91-080-22933099/22933503 (extn 107)
Fax: 91-080-23601428/23600085
Web: http://ces.iisc.ernet.in/energy
B.M. Jenkins Department of Biological and Agricultural Engineering, University of California Davis, California 95616, USA
Indu Shekhar Thakur School of Environmental Sciences, Jawaharlal Nehru University, New Delhi 110067, India
Citation:Bharath H. Aithal, S. Vinay, T. V. Ramachandra, 2018. Simulating urban growth by two state modelling and connected network. Modeling Earth Systems and Environment. © Springer Nature Switzerland AG 2018. Received: 30 April 2018 / Accepted: 11 August 2018. https://doi.org/10.1007/s40808-018-0506-1
|